MAIR: Multi-view Attention Inverse Rendering
with 3D Spatially-Varying Lighting Estimation
CVPR 2023
- JunYong Choi 1,2
- SeokYeong Lee 1,2
- Haesol Park 1
- Seung-Won Jung 2
- Ig-Jae Kim 1,3,4
- Junghyun Cho 1,3,4
- 1Korea Institute of Science and Technology(KIST)
- 2Korea University
- 3AI-Robotics, KIST School, University of Science and Technology
- 4Yonsei-KIST Convergence Research Institute, Yonsei University
Overview
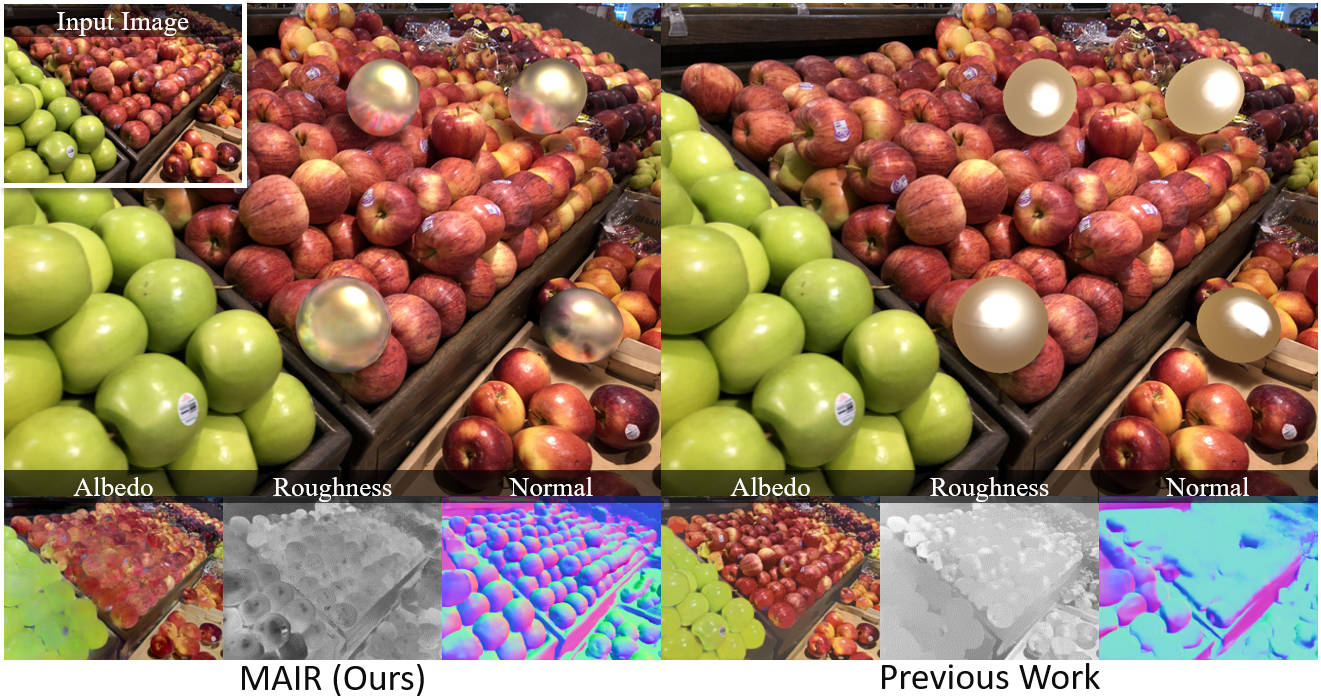
We propose a scene-level inverse rendering framework that uses multi-view images to decompose the scene into geometry, a SVBRDF, and 3D spatially-varying lighting. Because multi-view images provide a variety of information about the scene, multi-view images in object-level inverse rendering have been taken for granted. However, owing to the absence of multi-view HDR synthetic dataset, scene-level inverse rendering has mainly been studied using single-view image. We were able to successfully perform scene-level inverse rendering using multi-view images by expanding OpenRooms dataset and designing efficient pipelines to handle multi-view images, and splitting spatially-varying lighting. Our experiments show that the proposed method not only achieves better performance than single-view-based methods, but also achieves robust performance on unseen real-world scene. Also, our sophisticated 3D spatially-varying lighting volume allows for photorealistic object insertion in any 3D location.
Pipeline
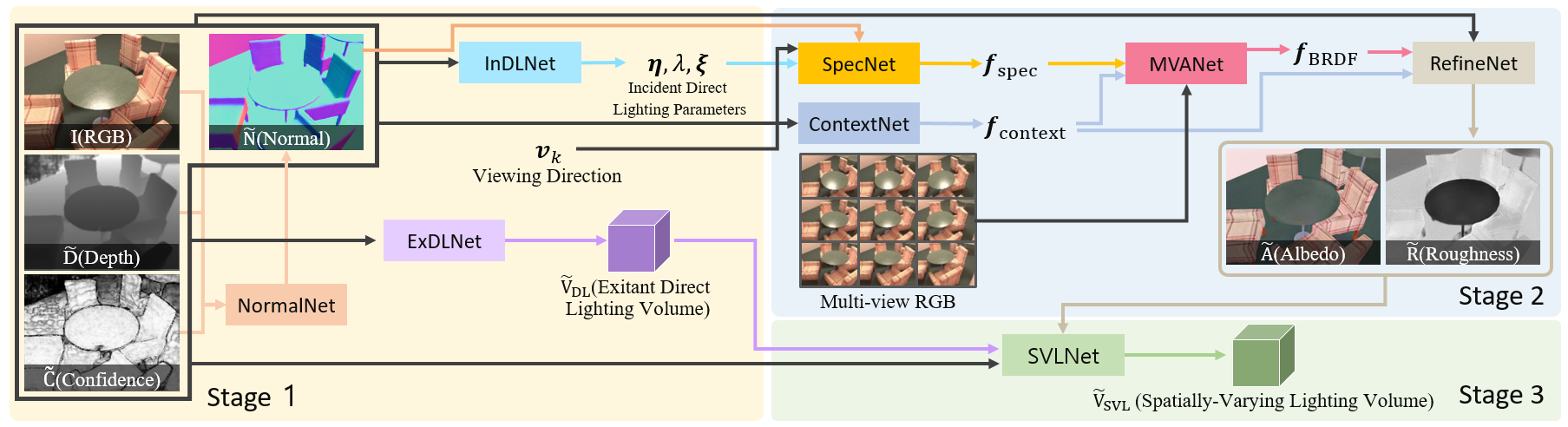
Comparisons
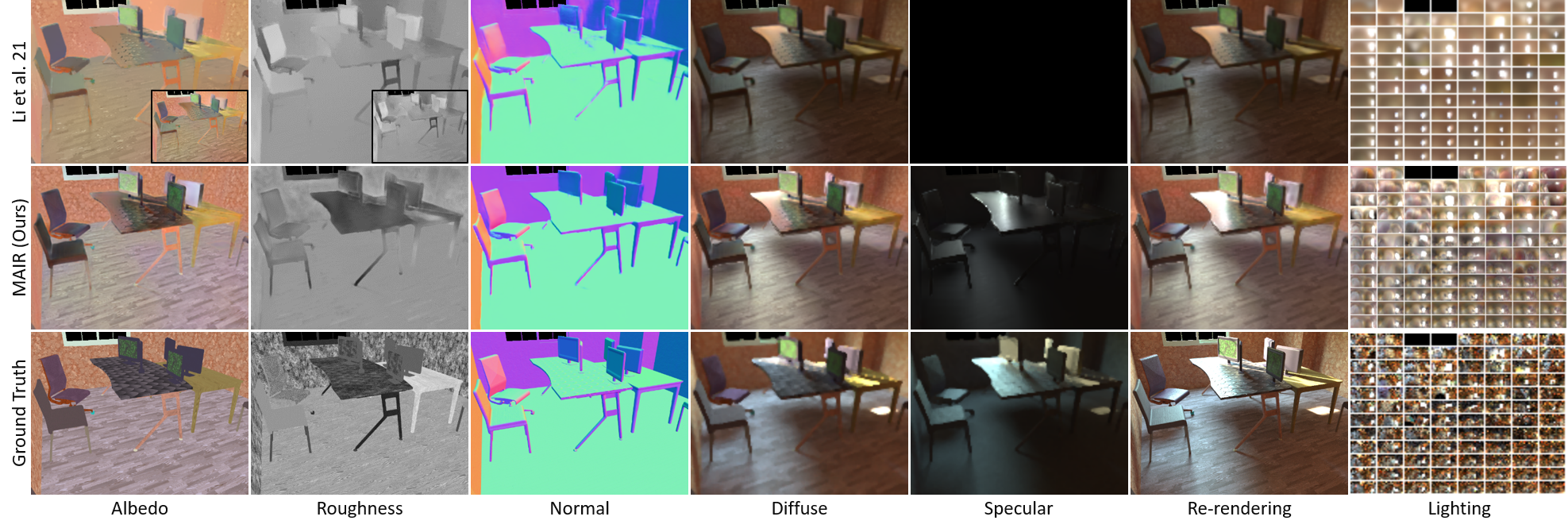
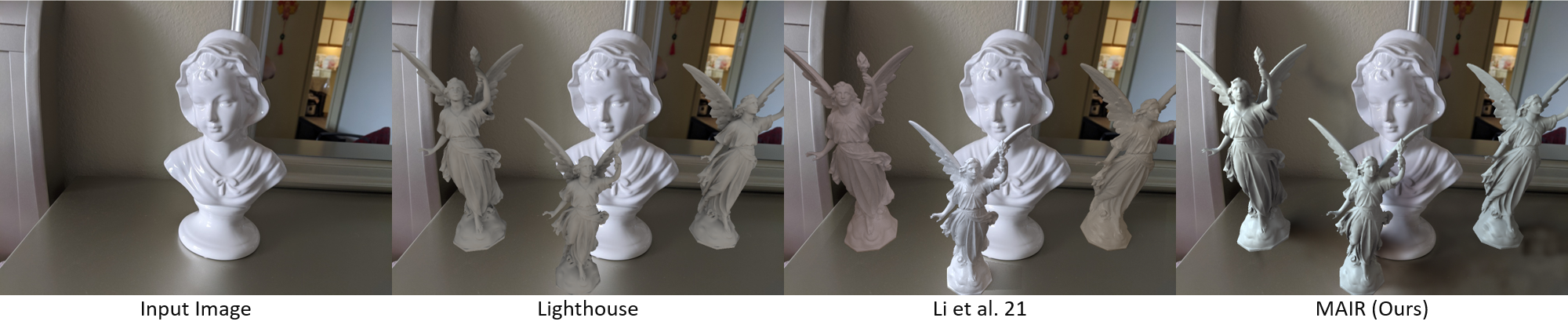

Object Insertion
View Synthesis + Chrome Sphere Insertion
Acknowledgments
This work was partly supported by Institute of Information & communications Technology Planning & Evaluation (IITP) grant funded by the Korea government(MSIT)(No.2020-0-00457, 50%) and KIST Institutional Program(Project No.2E32301, 50%).We have a special thanks to Zhengqin Li for helping us create OpenRooms FF dataset.
Images used for object insertion were imported from IBRNet. The view synthesis results were created using DirectVoxGo. The website template was borrowed from Michaƫl Gharbi and OpenRooms (Zhengqin Li).